Big data analytics is the use of advanced analytic techniques against very large, diverse data sets that include structured, semi-structured and unstructured data, from different sources, and in different sizes from terabytes to zettabytes.
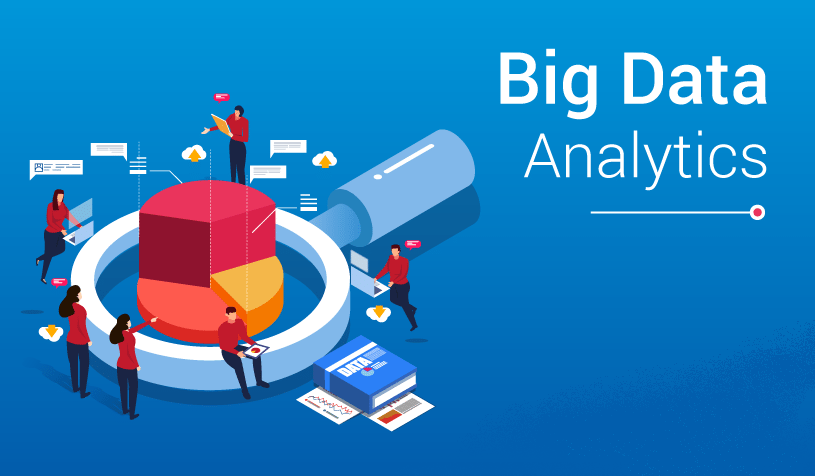
Use cases for big data analytics
>Improve customer integrations
Aggregate structured, semi- and unstructured data from touch points your customer has with the company to gain a 360-degree view of your customer’s behavior and motivations for improved tailored marketing. Data sources can include social media, sensors, mobile devices, sentiment and call log data.
>Detect and mitigate fraud
Monitor transactions in real time, proactively recognizing those abnormal patterns and behaviors indicating fraudulent activity. Using the power of big data along with predictive/prescriptive analytics and comparison of historical and transactional data helps companies predict and mitigate fraud.
>Drive supply chain efficiencies
Gather and analyze big data to determine how products are reaching their destination, identifying inefficiencies and where costs and time can be saved. Sensors, logs and transactional data can help track critical information from the warehouse to the destination.
Making Sense of Big Data: A Day in the Life of an Enterprise Architect
The rise of cloud storage — and the demand for remote, cloud-based access — has transformed the role of the enterprise data architect. Instead of simply managing an in-house database, architects now need to handle a host of new challenges related to data integrity, security, integration and analysis.
This Enterprise Management Associates (EMA) white paper reviews the challenges that confront an enterprise architect, including new data sources, more data projects, and more data platforms.
The authors explain how enterprise architects can nurture an in-house approach that’s able to handle these challenges, including:
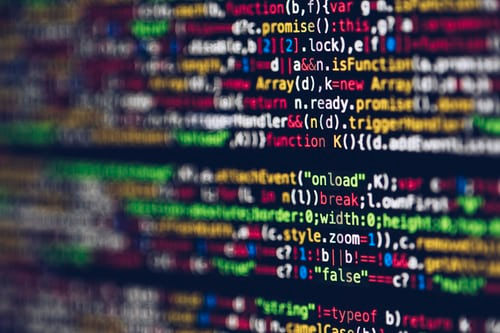
Using customizable applications to make implementations faster
Training internal staff instead of hiring expensive contractors
Collaborating closely with business stakeholders to help align data strategy with business needs
Deploying a hybrid data ecosystem to provide the foundation for self-service projects
CONCLUSION
The availability of Big Data, low-cost commodity hardware, and new information management and analytic software have produced a unique moment in the history of data analysis. The convergence of these trends means that we have the capabilities required to analyze astonishing data sets quickly and cost-effectively for the first time in history. These capabilities are neither theoretical nor trivial. They represent a genuine leap forward and a clear opportunity to realize enormous gains in terms of efficiency, productivity, revenue, and profitability.
The Age of Big Data is here, and these are truly revolutionary times if both business and technology professionals continue to work together and deliver on the promise.
Thank you for taking the time to read our book and we hope you enjoyed reading it as much as we did writing it. We’d like to conclude with a transcript from one of the most charismatic speakers on the Big Data circuit, Google’s Avinash Kaushik, from his presentation at Strata 2012, “A Big Data Imperative: Driving Big Action”:
I actually don’t really care about the promise of data unless they can deliver on that promise that comes with the data.
I’m going to try and bring things back to a practical level and open my talk with this quote, “Information is powerful,” said a Kenyan farmer, “it’s what we use it for that will define us.” I love, I love this quote. If you look at all the hype that’s connects to big data it solves for the first problem, if you listen .

Kommentarer