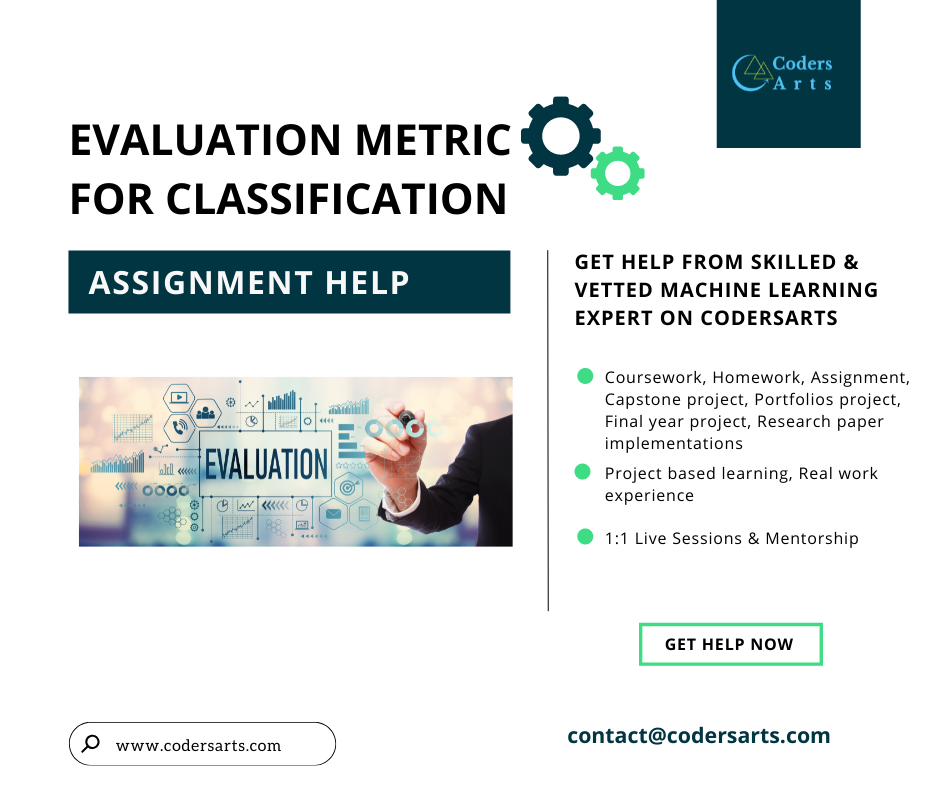
Need help with Evaluation Metric for Classification Assignment Help or Evaluation Metric for Classification Project Help? At Codersarts we offer session with expert, Code mentorship, Code mentorship, Course Training, and ongoing development projects. Get help from vetted Machine Learning engineers, mentors, experts, and tutors.
Are you stuck with your assignment? Are you looking for help in Evaluation Metric for Classification Assignment Help? Are you looking for an expert who can help in your assignment? We have an expert team of Data science professionals who would be available to work on Evaluation Metric for Classification Assignment. Our team will understand the requirements and will complete the assignment flawlessly and plagiarism free. Our expert will assure you that you will provide the best solutions for your assignment. Our Evaluation Metric for Classification Assignment help experts write the assignment according to the requirement given by the professor and by thoroughly following the university guidelines. Our expert will help you secure higher grades in the examination. We will complete the assignment before the time span with the best solution. Our Evaluation Metric for Classification Assignment help expert will provide the proper guidance and complete solution for your assignment.
Evaluation Metric for Classification
Evaluation metrics are related to the machine learning task. There are different types of metrics available for classification tasks such as precision-recall that are useful for multiple tasks. Classification are the example of supervised learning. By using the different metrics for performance evaluation, we can improve the accuracy of our model. When a Machine Learning model is deployed on unseen data without performing a proper evaluation utilising several evaluation metrics and relying just on accuracy, it might cause problems and result in bad predictions.
Classification is the process of predicting class labels from input data. There are just two possible output classifications in binary categorization. There can be more than two possible classes in multiclass classification.
Metrics
Accuracy : It counts how many positive and negative observations were accurately categorised. The number of correct predictions divided by the total number of forecasts is known as accuracy.
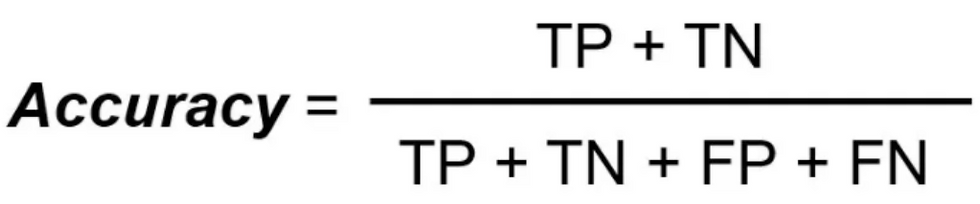
On unbalanced problems, accuracy should be avoided. Then, just classifying all observations as the majority class makes it simple to achieve a high accuracy score.
Confusion Matrix : The Confusion Matrix is a performance metric for machine learning classification tasks with two or more classes as an output. It's a table of predicted and actual values.
A confusion matrix is defined as the table that is often used to describe the performance of a classification model on a set of the test data for which the true values are known.
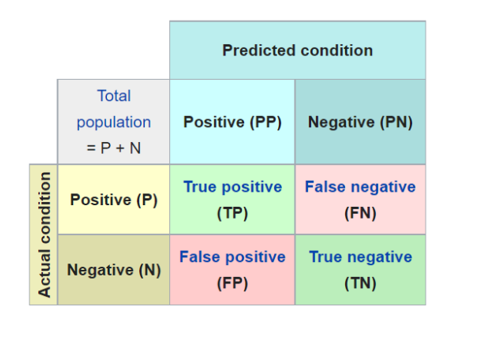
Suppose two possible classes, yes and no. if we were predicting the presence of disease, for example yes would mean they have disease and no means they don't have disease
True positive TP : predicted yes and they do have the disease
True negative TN : predicted no they don't have the disease
False Positive FP : predicted yes, but they don't actually have the disease
False Negative FN : predicted no, but they actually do have the disease
Precision : Precision is the ratio of true positive and total positive prediction. When model makes many incorrect positive classification, or few positive predictions, this increase the denominator and makes the precision small

Recall : It is a true positive rate. It measures correctly identifying true positives for the model. It tells us about all positive data points.
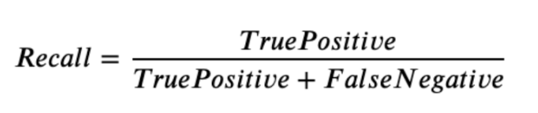
F1 Score :It’s the harmonic mean between precision and recall.

It would be effective in the following case :
When FP and FN are equally costly
Adding more data does not effectively change the outcome
True negative is high
AUC-ROC : The receiver operator characteristic (ROC) is a probability curve that plot the TPR against the FPR at various threshold value
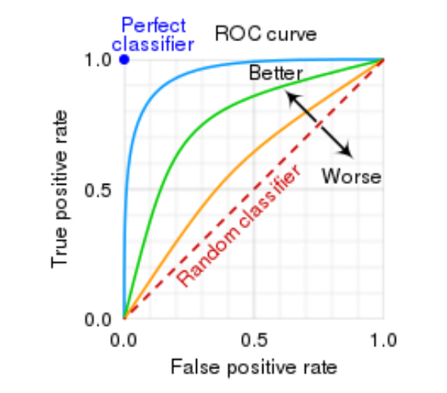
How Codersarts can Help you in Evaluation Metric for Classification Assignment ?
Codersarts provide:
Evaluation Metric for Classification Assignment help
Evaluation Metric for Classification Project Help
Mentorship in Evaluation Metric for Classification from Experts
Evaluation Metric for Classification Development Project
If you are looking for any kind of Help in Evaluation Metric for Classification Assignment or Evaluation Metric for Classification project Contact us
Comments